Artificial intelligence (AI) is gaining momentum, and its importance for the future of work in many areas, such as medicine and banking, is continuously rising. However, insights on the effective collaboration of humans and AI are still rare. Typically, AI supports humans in decision-making by addressing human limitations.
However, it may also evoke human bias, especially in the form of automation bias as an over-reliance on AI advice. We aim to shed light on the potential to influence automation bias by explainable AI (XAI). In this pre-test, we derive a research model and describe our study design. Subsequentially, we conduct an online experiment with regard to hotel review classifications and discuss first results. We expect our research to contribute to the design and development of safe hybrid intelligence systems.
Introduction
Over the last years, artificial intelligence (AI) has made significant progress (Lecun et al. 2015) and is being more and more integrated into standard applications and, thus, is becoming commoditized. However, especially in high-stake decision-making environments—such as medicine or banking—fully autonomous AI may be neither desirable nor (legally) possible. Additionally, AI capabilities are often limited to narrowly defined application contexts as the utilized algorithms often struggle to handle instances that differ from the patterns learned during training (D’Amour et al. 2020). In these cases, humans can contribute and leverage their unique capabilities such as intuition, creativity, and common sense. This line of thought gives rise to the vision of so-called hybrid intelligence (Dellermann et al. 2019), proposing to combine the complementary capabilities of humans and AI.
While AI has many advantages (like the capability to process significant data volumes), it may evoke human bias that negatively affects the outcome of hybrid intelligence (Challen et al. 2019). Specifically, automation bias (AB) is the “tendency to use automated cues as a heuristic replacement for vigilant information seeking and processing” (Mosier and Skitka 1999)—essentially representing an overreliance on AI advice.
Automation bias may trigger severe consequences
Automation bias may trigger severe consequences as illustrated by human drivers directing their cars into rivers when blindly adopting erroneous routing advice (Santiago 2019). Similarly, Alberdi et al. (Alberdi et al. 2004) report that oncologists interpreting mammograms are accepting incorrect system advice in 33% to 40% of all cases—with serious impacts for their patients. Schemmer et al. /Explainable AI and Automation Bia Thirtieth European Conference on Information Systems (ECIS 2022), Timisoara, Romania 2 Research on AB in AI-based decision-making is still rare in IS research (Jussupow et al. 2021).
This is plausible for two reasons: First, in the nascent, rather explorative phase of AI with often low AI performance, under-reliance was the more prominent issue. Second, prior work in IS often just assumed
system advice to be perfectly correct (Jussupow et al. 2021). Thus, humans should always agree with the AI, and an issue of over-reliance could not arise per definition. However, with the increasing commoditization of AI and its application in more high-stake areas both have upped its performance as well as the realization of its imperfection. So, both understanding AB and design mechanisms to avoid it enjoy increasing research interest and practical relevance (Jussupow et al. 2021; Liel and Zalmanson 2020).
Basic understanding of the data
To address AB, humans should be enabled to have a basic understanding of the data, algorithms, and
capabilities that determine the AI advice (Dzindolet et al. 2003). These insights would help them to
identify incorrect AI advice (Arora 2020). The emerging research stream of explainable AI (XAI) (Bauer
et al. 2021) aims to equip human users with such insight into AI decisions. This might help the human
decision-maker to better judge the quality of an AI advice. An analogy would be the interaction between
a management consultant, who provides advice, and his client as the decision-maker. To assess the
quality of the recommendation, the decision-maker will ask the consultant to describe the reasoning.
Based on the explanations, the decision-maker should be able to determine whether the advice can be
relied upon or not. The same logic should hold for an “AI consultant”. Explanations, thus, can also be
seen as discrimination support for the decision-maker to assess whether to rely on the AI.
On the other hand, experimental studies indicate that explanations in human-AI collaboration can lead
to “blind trust”, i.e. following the AI advice without any evaluation of its trustworthiness (Bansal et al.
2021; Zhang et al. 2020). Research shows that explanations are sometimes interpreted more as a general
sign of competence (Buçinca et al. 2021) and have a persuasive character (Bussone et al. 2015). This is
supported by psychology literature which has shown that human explanations cause listeners to agree
even in the presence of wrong explanations (Koehler 1991). The same effect could occur when the
explanations are generated by AI. This potential effect of explanations on AB has severe consequences
for the design of human-AI systems, especially ifstatus-quo explanations are mostly seen as meaningful.
The ambiguous trade-off between enabling the humans to discriminate the AI’s advice, thereby reducing
AB, and on the other hand, the tendency that the sole existence of an explanation could increase the
reliance of the human on the AI which increases AB needs to be studied thoroughly. We, therefore,
intend to shed light on the opposing effects that XAI may exert on AB by asking:
RQ: How does explainable artificial intelligence affect automation bias?
Research model of 5 hypotheses
To answer this question, we propose a research model, including five hypotheses, and design an online experiment to test our hypotheses. In a between-subject study, participants classify whether hotel reviews are genuine or fake, with the support of an AI—once without and once with explanations. First results of our pre-test with a limited number of participants indicate that explanations tend to increase AB. In the next step of our work, we intend to run our main study with sufficient participants to derive more insights. While certainly concrete tradeoffs of explanation effects on AB hinge on the domain and type of explanations, even our preliminary results hint towards the popular (and often undisputed) call that XAI may come at a price—the over-reliance on AI-based decisions.
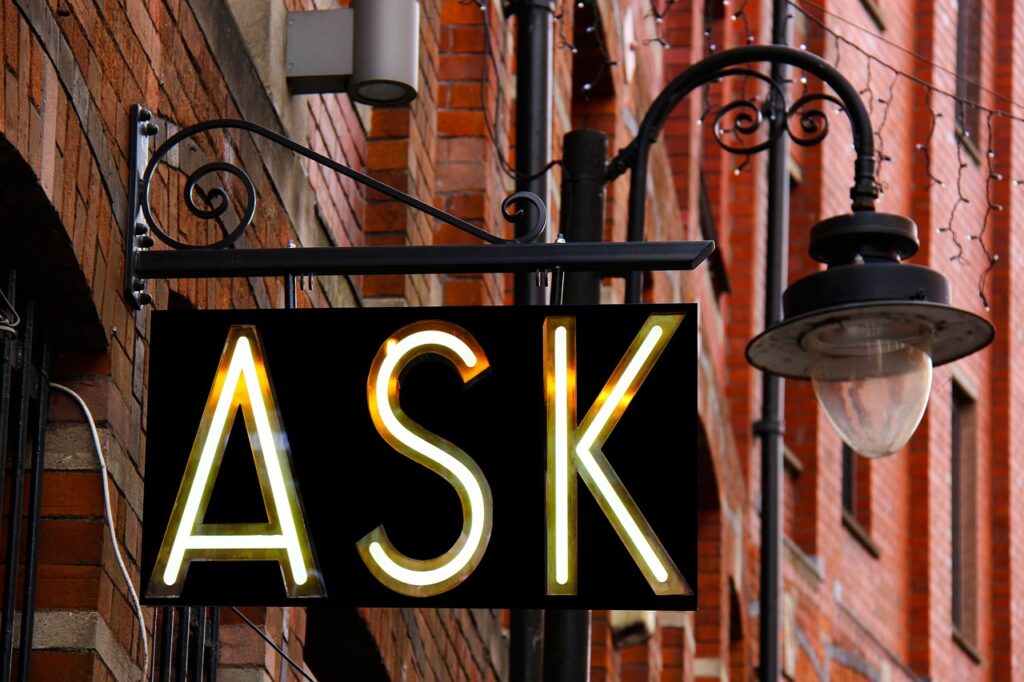
With our work, we take a new perspective on XAI affecting automation bias. By providing initial insights into XAI and AB, we contribute to advancing the research field of hybrid intelligence. We expect our work to have concrete managerial implications informing the design of hybrid intelligence systems, especially for critical scenarios, such as medical diagnoses.
Discussion and Expected Contribution
In this article, we describe a first pre-test regarding the influence of XAI on automation bias, an over reliance on AI. To investigate the effect in-depth, we first derive a research model, including hypotheses, and then conduct an online experiment. In the online experiment, we let participants classify hotel reviews as fake and genuine. The results of our study indicate that explanations of AI increase automation bias.
Studies have shown that, in particular cases, significant differences exist between the occurrence of commission and omission errors (Lyell and Coiera 2017). In line with these studies, we find differences
between AI and XAI commission errors (still not significant), but no differences in terms of omission errors. One interpretation would be that humans are more likely to classify a review erroneous as fake, i.e., humans tend to believe the AI more if the recommendation detects a state of interest.
Although certainly effects of explanation on automation bias depend on the domain and type of explanations, our results illustrate that XAI may increase over-reliance on AI advice. While one could argue that this is because of confusing state-of-the-art explanations , which is indicated by the qualitative feedback, we believe the underlying reason is far more complex. If the explanations are confusing, it is imminent that humans would have a hard time detecting when the AI is correct.
Conclusions
However, it is not intrinsically logical that a confusing explanation increases automation bias. Confusing explanations could also lead to an aversion. Therefore, the underlying mediators of automation bias need to be rigorously examined to understand the relationship between explanations and automation bias.
In terms of the mediators of automation bias in our current study, we do not find any differences between
trust in the AI and XAI setting within our limited pre-test. However, we find a non-significant difference in terms of MM. Participants in the AI treatment have reported a higher MM goal. This could mean that humans find it challenging to build a MM of the AI, due to the confusing explanations. Additionally, we conducted a correlation analysis to provide first insights on how XAI influences automation bias.
We find no significant results but an indication of the direction of the effects. Trust seems to increase
automation bias while a positively influenced MM seems to decrease automation bias which is both in line with our hypotheses. Thus the negative effect of XAI on automation bias in our study could be caused by a negative influence of XAI on the human MM.
In future work, we plan to analyze the influence of explanations on automation bias in-depth. More specificially, in our main study, we plan to investigate the mediation effects more in depth by conducting
mediaton analysis (MacKinnon et al. 2007) and structural equation modeling (Ullman and Bentler 2012). By analyzing the mediators of automation bias, we aim to develop further insights into automation bias and thereby create the best possible human-AI collaboration. Despite being a first pretest, we already contribute to the body of knowledge. We derive a research model and an experimental design to investigate the influence of XAI on automation bias and report as well as discuss first preliminary results.
With the commoditization of AI, automation bias will become a serious issue that needs to be analyzed. It must be emphasized that currently explanations of AI always have a positive connotation and are even anchored in law, such as in the European right of explainability (Bauer et al. 2021). Our research indicates that explanations could lead to over-reliance and thus have serious consequences.
Read more…
19 April 2022
Authors: Max Schemmer, Niklas Kühl, Carina Benz, Gerhard Satzger
This article has been extracted and freely adapted from Arxiv-Cornell University